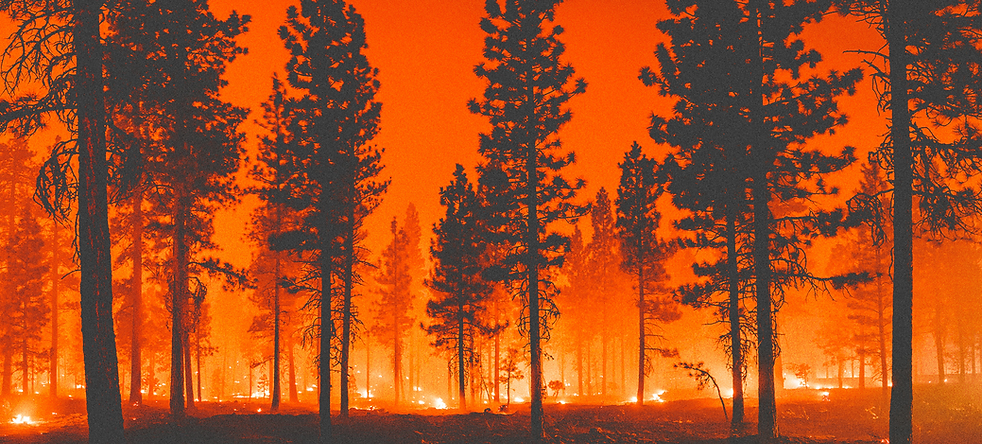
Geographically Weighted Regression Analysis of Wildfires in British Columbia
Joshua Green

RESOURCES
Software and Tools
-
ArcGIS Pro
-
QGIS
-
Jupyter Notebook
-
MGWR 2.0 Software
BIBLIOGRAPHY
Bush, E., & Lemmen, D. S. (Eds.). (2019). Canada's changing climate report. Government of Canada= Gouvernement du Canada.
Flannigan, M., Cantin, A. S., De Groot, W. J., Wotton, M., Newbery, A., & Gowman, L. M. (2013). Global wildland fire season severity in the 21st century. Forest Ecology and Management, 294, 54-61.
Gillett, N. P., Weaver, A. J., Zwiers, F. W., & Flannigan, M. D. (2004). Detecting the effect of climate change on Canadian forest fires. Geophysical Research Letters, 31(18).
Kirchmeier‐Young, M. C., Gillett, N. P., Zwiers, F. W., Cannon, A. J., & Anslow, F. S. (2019). Attribution of the influence of human‐induced climate change on an extreme fire season. Earth's Future, 7(1), 2-10.
Kochtubajda, B., Stewart, R. E., Flannigan, M. D., Bonsal, B. R., Cuell, C., & Mooney, C. J. (2019). An assessment of surface and atmospheric conditions associated with the extreme 2014 wildfire season in Canada’s Northwest Territories. Atmosphere-Ocean, 57(1), 73-90.
Koutsias, N., Martínez-Fernández, J., & Allgöwer, B. (2010). Do factors causing wildfires vary in space? Evidence from geographically weighted regression. GIScience & Remote Sensing, 47(2), 221-240.
Li, Z., & Fotheringham, A. S. (2020). Computational improvements to multi-scale geographically weighted regression. International Journal of Geographical Information Science, 34(7), 1378-1397.
Martínez-Fernández, J., Chuvieco, E., & Koutsias, N. (2013). Modelling long-term fire occurrence factors in Spain by accounting for local variations with geographically weighted regression. Natural Hazards and Earth System Sciences, 13(2), 311-327.
Maudlin, L. C., Wang, Z., Jonsson, H. H., & Sorooshian, A. (2015). Impact of wildfires on size-resolved aerosol composition at a coastal California site. Atmospheric Environment, 119, 59-68.
Monjarás-Vega, N. A., Briones-Herrera, C. I., Vega-Nieva, D. J., Calleros-Flores, E., Corral-Rivas, J. J., López-Serrano, P. M., ... & Jolly, W. M. (2020). Predicting forest fire kernel density at multiple scales with geographically weighted regression in Mexico. Science of The Total Environment, 718, 137313.
Oliveira, S., Pereira, J. M., San-Miguel-Ayanz, J., & Lourenço, L. (2014). Exploring the spatial patterns of fire density in Southern Europe using Geographically Weighted Regression. Applied Geography, 51, 143-157.
Partain Jr, J. L., Alden, S., Bhatt, U. S., Bieniek, P. A., Brettschneider, B. R., Lader, R. T., ... & Ziel, R. H. (2016). 4. An Assessment of the role of anthropogenic climate change in the Alaska fire season of 2015. Bulletin of the American Meteorological Society, 97(12), S14-S18.
Su, Z., Hu, H., Tigabu, M., Wang, G., Zeng, A., & Guo, F. (2019). Geographically weighted negative binomial regression model predicts wildfire occurrence in the Great Xing’an Mountains better than negative binomial model. Forests, 10(5), 377.
Tett, S. F., Falk, A., Rogers, M., Spuler, F., Turner, C., Wainwright, J., ... & Lehmann, C. E. (2018). Anthropogenic forcings and associated changes in fire risk in Western North America and Australia during 2015/16. Bulletin of the American Meteorological Society, 99(1), S60-S64.
Zhang, Z. X., Zhang, H. Y., & Zhou, D. W. (2010). Using GIS spatial analysis and logistic regression to predict the probabilities of human-caused grassland fires. Journal of arid environments, 74(3), 386-393.
Zhang, H., Han, X., & Dai, S. (2013). Fire occurrence probability mapping of northeast China with binary logistic regression model. IEEE Journal of Selected Topics in Applied Earth Observations and Remote Sensing, 6(1), 121-127.